Necessity is the mother of invention
Here are some thoughts on R1, the model that’s got everybody’s panties in a bunch. I’ve written it in simple bullet points to make it easier to consume and easier for me to write quickly. I explore what R1 means for the AI marketplace and how much significance we should give to the moment. Spoiler alert: I think the market’s response on January 27th was a major overreaction.
Many of my clients contacted me in the last 48 hours asking for my perspective on China’s new AI model, DeepSeek R1, and the resulting fallout in the market. I covered R1 in the most recent issue of Synthetic, my weekly AI newsletter.
Here are some thoughts on R1, the model that’s got everybody’s panties in a bunch. I’ve written it in simple bullet points to make it easier to consume and easier for me to write quickly. I explore what R1 means for the AI marketplace and how much significance we should give to the moment. Spoiler alert: I think the market’s response on January 27th was a major overreaction.
DeepSeek R1 is impressive
According to DeepSeek-supplied benchmarks, R1’s performance is comparable with OpenAI’s o1 model, though lower performance than OpenAI’s latest flagship o3 model.
R1 is quick, churning out 60 tokens per second.
Like OpenAI’s o1 model, R1 uses chain-of-thought reasoning to solve challenging problems. Unlike o1, R1 fully exposes its chain of thought, so you can see the steps it’s using to ‘think’ through challenges.
It took just 60 days to train the model. It was likely trained on just 2048 H800 Nvidia GPUs, though nobody knows with certainty what it was trained on, and DeepSeek’s PR department isn’t responding to press inquiries.
The model is said to have cost less than $6 million to create. That’s roughly 1/20th the cost of building OpenAI’s current workhorse model, GPT-4o, which was trained on more powerful H100 GPUs, perhaps using as many as 100,000 chips for training.
R1 uses several innovations to achieve its impressive results:
* R1 was trained using lower precision 8-bit floating point (FP8) and uses an efficient E4M3 format on all tensor calculations. This reduces the memory needed by 75% and speeds up training.
* A new multi-token system can process entire phrases simultaneously, speeding up model inference. This approach does reduce accuracy, but it doubles token throughput.
* R1 is built as a system of specialists, with multiple sub-models stitched together. Only parts of the model are used at any given time, reducing energy consumption and improving speed. This approach is not new, but R1 has apparently implemented it well.
There's a more detailed analysis of what DeepSeek actually did to achieve the R1 breakthrough here. DeepSeek achieved all this with a team of only 200 people.
What was the fallout?
The release of DeepSeek R1 wiped almost $600 billion off Nvidia’s market cap in one day, as the stock closed down 17%. According to CNBC, this was the greatest total stock price drop by a company in the stock market's history.
Other tech stocks took major tumbles. The tech-heavy NASDAQ index was down 3% on the day.
Meta’s rumored to be in panic mode and may throw out its work on Llama 4.0 due to the DeepSeek R1 release. We can only assume that R1 outperforms Llama 4.0 (which was due to be released later this quarter), and Meta engineers are now trying to figure out what they can do to correct the course.
Commentators have declared this a huge moment for the AI sector. It is certainly a moment that should cause great introspection in the AI research community, and we should all give it the attention it deserves, but there’s a lot of hyperbole being thrown around. Peter Diamandis commented that “Nvidia’s moat has turned into a puddle" and Marc Andreessen called this “China’s Sputnik moment” for AI.
DeepSeek R1 has shortcomings and issues that may mean you don’t want to use it:
The model was created by a Chinese company with ties to the Chinese Communist Party and will reflect a particular ideology. Some users have reported that the model becomes ‘busy’ when asked questions about Tiananmen Square or soft pedals answers about what happened in Beijing in 1989.
The multi-token approach used in R1 might double throughput but it comes at a cost of reduced accuracy.
The U.S. government will not approve applications that use it and will not be CUI (Controlled Unclassified Information) approved.
DeepSeek’s iOS app users have found that the R1 app is logging keystrokes and sending data back to China. It has to send data to the cloud-hosted model for the app to work, of course, but we must be clear-eyed about where our data is going. It’s going to China.
Be sure to apply critical thinking when you see posts online claiming that DeepSeek is "amazing!!"
What can we learn from R1?
The release of DeepSeek’s R1 model smashes two previously held notions:
1. The Chinese are significantly behind Western AI researchers.
2. Open-source models lag closed-source model performance by half to an entire generation.
R1 is cheaper to use and is available as open source, so anyone can use it (if they have the hardware needed to run it) at low cost. All AI research labs are constantly improving their models to make them run cheaper and faster. Model costs have reduced by 99% over the last two years, and I expect this process to continue. The techniques used in R1 (which other AI players will adopt or use to inspire further innovation) will only accelerate this process.
The Chinese are often mischaracterized as only being good at copying the work of others. Hopefully, R1 dispels that rumor and reinforces our understanding that China has capable engineers and researchers who can deliver performance close to that of the leading frontier models from Western AI research labs.
While DeepSeek R1 offers some new ideas on how to produce better models that are cheaper to build and operate, it doesn’t signal the end of Nvidia. Far from it.
What’s my analysis?
Short-term panic will lead to medium- and long-term benefits for the entire industry. AI research labs have all read the paper, are analyzing R1, and will build on that work in their next generation of models. This will take performance and capability levels even higher.
If future models are cheaper and easier to develop as a result of adopting some of the approaches DeepSeek claims to have used, it will make AI more accessible to more people and increase usage. The internet has been filled with mentions of Jevons Paradox, and I think that’s appropriate. The cheaper AI is to use, the more it will be used. Overall, that will lead to more net demand for AI (and increased demand for AI chips, infrastructure, and data centers), not less. I believe R1 is actually good news for Nvidia and the other tech giants, not bad. It’s why I bought NVDA on the dip.
DeepSeek R1 is a single data point that's part of a much longer race. It won’t be long before Google, Anthropic, or another frontier lab releases a model that exceeds R1’s capabilities in every way (cost, performance, intelligence, etc.) That said, DeepSeek has established itself as an impressive team of talent that may continue to disrupt the AI space. And it’s not done yet. Their new Janus-Pro model is said to outperform Stable Diffusion and OpenAI’s DALL-E 3 models. We should all keep an eye on what they do next.
There will be a response from the U.S. government. The last thing they want is China taking AI leadership and millions of Americans loading a Chinese model on their phones for daily use. We’ve seen this movie before with Tik Tok. With DeepSeek at the top of the app charts, we should expect additional export controls and other responses from the U.S. government in the coming days and weeks.
Summary and conclusions
Overall, the emergence of R1 as a worthy competitor is good for the AI industry, will accelerate AI development, and could be better for the planet. AI researchers will take inspiration from DeepSeek’s approach and find leaner, meaner ways to develop future models that make better use of the computing power they have at their disposal.
Size still matters. Future AI advances will come from scaling AND innovation. R1 is an example of innovation, but it does not negate the value of scaling. Models will continue to benefit from extreme computing power for training and inference. If you gave DeepSeek researchers 10x the computing capability they use to develop R1, I am confident their next model would outperform it.
If AI researchers, inspired by R1, build more streamlined models that consume less energy to train and use, we may need less energy to run the models of the future. This is better for climate change and may accelerate the path to AGI in a compute-constrained environment.
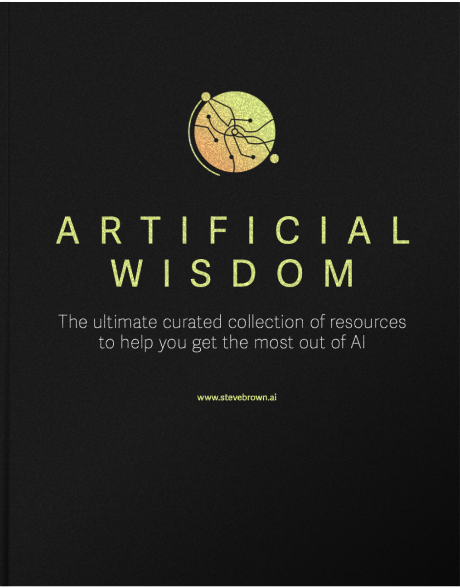
The unlimited curated collection of resources to help you get the most out of AI
#1 AI Futurist
Keynote Speaker.
Boost productivity, streamline operations, and enhance customer experience with AI. Get expert guidance directly from Steve Brown.
.avif)
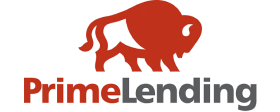
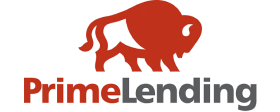
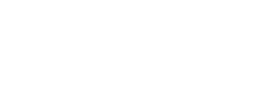
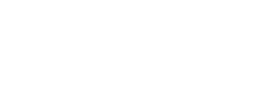